Artificial intelligence is becoming increasingly vital in everyday life, impacting decision-making, education, and transportation, including driving cars. It's gradually replacing many conventional methods across various sectors. In industries like manufacturing, where traditional approaches such as lean manufacturing have long been used to optimize processes, manufacturing companies are now using computer vision (CV) based AI systems to gain visual insights into the work. An example of this is, how i-5O has replaced the andon light system with an improved CV based real-time alerts.
Regular maintenance becomes crucial to fully utilize the benefits of these computer vision systems. Without this upkeep, unforeseen issues can quickly transform a good model into a liability. This highlights the significance of why the model fails and how we can actively monitor CV-based systems to ensure their continued effectiveness and reliability.
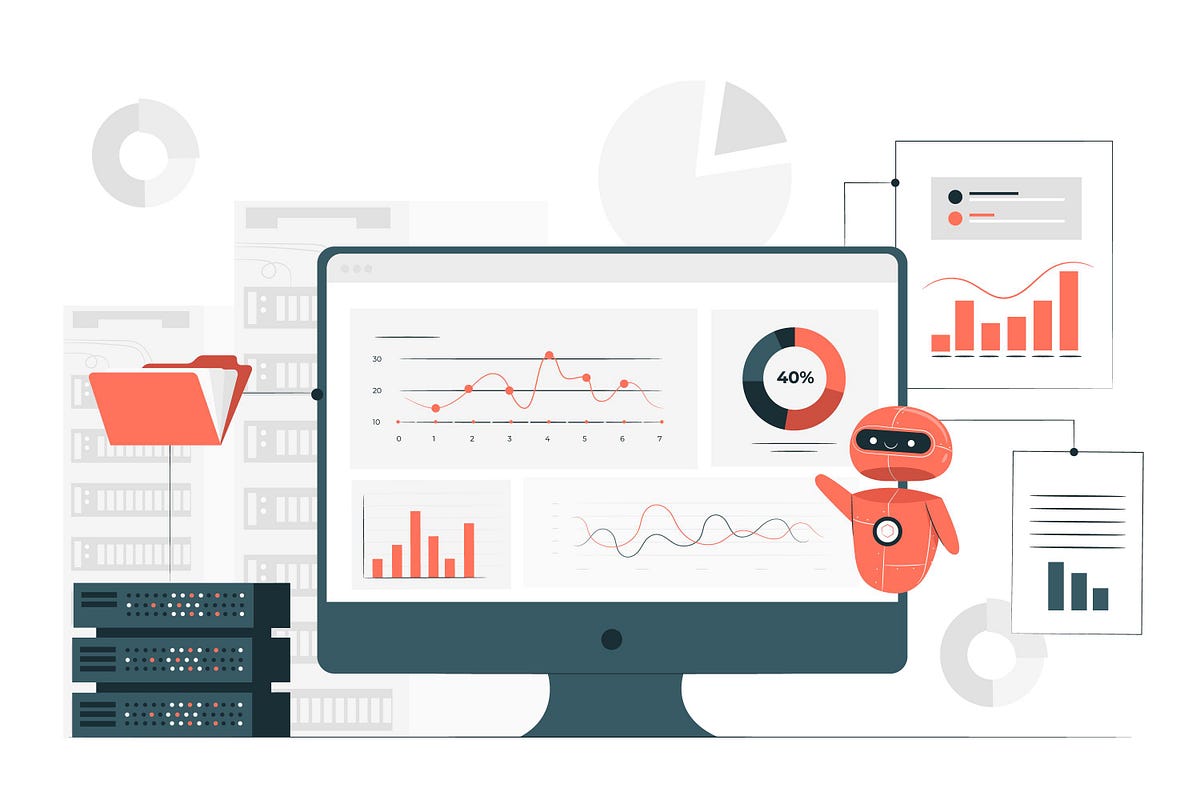